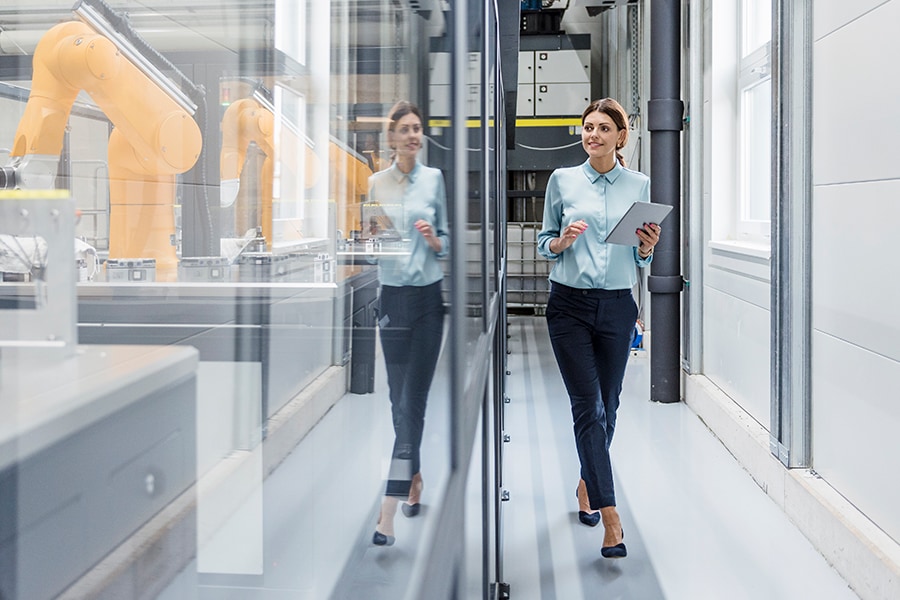
Unlock the full potential of analytics and artificial intelligence
PwC’s Analytics & AI Transformation Solution
Learn moreArtificial intelligence (AI) has accelerated innovation across industries, in the process reinventing the way we do business. But what happens when an organization’s governance practices don’t evolve in tandem with its AI initiatives?
That’s when facial recognition tools exhibit racial bias, autonomous vehicles go rogue and targeted ads violate civil rights law. Though these occasions show the increased reliance on AI technology to make critical decisions, they also highlight the need to manage AI risks and adopt responsible, ethical AI practices.
Artificial intelligence (AI) has accelerated innovation across industries, in the process reinventing the way we do business. But what happens when an organization’s governance practices don’t evolve in tandem with its AI initiatives?
That’s when facial recognition tools exhibit racial bias, autonomous vehicles go rogue and targeted ads violate civil rights law. Though these occasions show the increased reliance on AI technology to make critical decisions, they also highlight the need to manage AI risks and adopt responsible, ethical AI practices.
In response, academics, non-governmental organizations (NGOs) and some policymakers recommend the adoption of algorithmic impact assessments (AIAs).
Designed to evaluate the end-to-end AI life cycle, AIAs provide significant details on AI systems and their impact.
Impact assessments are nothing new for many companies. But to properly govern these diverse systems, assessments should be dynamic in structure and enable modifications that suit an organization’s specific environment.
There is no currently agreed-upon approach to impact assessments. However, one approach is to consider AIAs as extensions to data privacy impact assessments (DPIAs), which are commonly used to address data privacy concerns and to comply with the EU’s General Data Protection Regulation (GDPR). Supported by enhanced governance systems, these impact assessments evaluate potential benefits, risks and remediation processes.
When personal data is being processed, for instance, a privacy impact assessment can be triggered under GDPR. “Risky” data processing of personally identifiable information may require a DPIA. In some settings, AI has the ability to make non-personal data identifiable. Take a user’s social media “likes.” While not considered personal data under GDPR, “likes” can be used to assume a user’s gender, sexuality, age, race and political affiliations. In spite of this, current DPIAs may not be required for this use case, which points to the need for bridging the gap with an AIA.
Algorithmic impact assessments, which go even further than DPIAs are designed to achieve four main goals.
Because AIAs can be modeled from existing frameworks in data protection, privacy and human rights policies, they may represent an augmented assessment rather than an entirely new process. Impact assessments are likely not new to your organization, so you can use existing assessments as a foundation and build on that. As part of the process, you should ask relevant questions, including: What are the societal and reputational implications associated with not evaluating these cases? How can we conduct a thorough assessment of an AI system without overburdening organizations?
What’s essential to remember is that an AIA can provide essential details on AI systems and their impact, while also helping you manage AI risk and adopt responsible, ethical AI practices.
In our next blog, we’ll look more closely at the AI life cycle and how AIAs come into play.