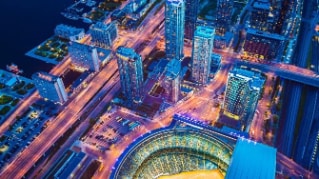
Redefining the future of work with Agentforce
PwC empowers organizations at every stage of their AI journey, delivering innovative customer experiences with AI-powered agents from Salesforce.
Matt Gretczko
Principal, PwC US
It’s hard to avoid the topic of AI as you scroll through news or social media posts daily. Though we have seen other technologies that have had as much disruption as AI, what seems to be readily apparent, is the speed at which AI, agents, and digital labor is evolving may be unmatched. It is improving exponentially.
Take even the semantics. Three months ago, we were just talking about “agents”. Now we are talking about digital labor. However, as we think about organizations and their desire to deploy this new form of labor, what we are starting to uncover is that the act of building an agent can become quite commoditized. This is not a bad thing, the technology is maturing so quickly that it does not require developers, or a deep technological background, to be able to create agents.
Yet, that poses an important and real question: where does the effort and work to stand them up come from, and how do you align it to drive value. As an early adopter of this technology for some customers, we have started to uncover the true areas of focus that are critical to make these agents, and the subsequent digital labor they provide, successful.
Anyone that has been in the technology space for some time, knows the importance of data. We’ve seen an evolution around the challenges with it as well. First (though not going back to the start of time), the issue was how to find new ways to structure data to make it usable. Then, it was moving data to the cloud which remains ongoing. Then, it was unlocking the data, interoperability, and taking on more API driven architectures. Now, it’s how can the data I’ve invested so heavily in drive agents, AI, and activation for my customers, employees, stakeholders, while also providing insights in parallel to help me evolve my business.
This is the first important step in building agents right, which can ultimately become effective digital labor. You should evaluate the data you have in your enterprise and spend the time understanding where that data sits, how it can be utilized, and whether it's in a structure and format to help drive the appropriate outcomes for the large language models (LLMs) and other tools interacting with it. Otherwise, you may soon find out that while the agents can react and respond, you may spend unnecessary time creating more complex instructions and guardrails to make up for the lack of appropriate data.
This includes assessing your overall data infrastructure and whether it's really built to support the change that is coming.
Developing agents requires one to not just build an agent generically, but build an agent based upon the appropriate knowledge and guidance within the industry, business, or function which you are operating. This is not to say that you can’t build agents and then redeploy them with minimal effort to solve other challenges. However, it does mean that if you are going to build an agent, you should factor in the implications of such agent. For example, if you were to build an agent for a large hospital system to allow patients to do autonomous self-scheduling — the reality is, you could stand this up rather quickly, but the question is “are the right patients scheduling”. To answer that question, you should factor in insights that you have around your capacity, your service lines, your growth goals, your knowledge of no-show rates, potentially even patient preferences, and other attributes. Meaning, it's not just good enough to create an agent that allows for scheduling, you want to be scheduling the right patients, for the right appointments or procedures, at the right facility, and at the right time.
To do this, you should either have, or leverage those that have, the industry insights to inform the agent with the appropriate combination of data, instructions, and guardrails to enable the desired outcome. Not to mention, this should strongly align to your Responsible AI framework to manage risks.
This one is pretty straightforward.
LLMs, both the volume and versioning, are evolving at an incredibly rapid pace. The belief is that while some may be able to solve a broad range of inquiries and problems, many are starting to be purpose fit — meaning, some are better at processing data, some are better at creating code, and others are better at creating images.
Regardless of their purpose and fit, the consideration here is that it is going to be difficult to stay apprised of every LLM, from every vendor, and where and how to pick the right one. So, the governance model and framework that allows your agents to have flexibility on which LLM they utilize but also allows for constant reevaluation of which is selected can be critical. This can enable you to effectively monitor and measure success and have a plan for evolving based on whether you are achieving the value that was identified. For the monitor component, this is incredibly important as unlikely traditional technology, agents can be continuously learning, so testing alone cannot meet the requirement. Monitoring can allow you to have real-time data to enable the constant learning of the agents themselves. The balance between when testing completes, and monitor commences can be a critical juncture in the deployment process.
Even more importantly with this technology, the ability to react quickly and effectively can be critical to enabling adaptability. This includes understanding why something went wrong, which may not just be the design of the technology.
As we think about the development of agents, and some of the topics above, we also do not want to forget about the experience. Just because an agent is “autonomous” doesn’t mean it’s a great experience. Take the analogy of telephony and how it moved to IVR type interactions, while it drove benefits for organizations and was more effective, most consumers felt it was a bad experience so that then transitioned to intent based calling which allowed for a more natural way to route a caller.
Same thing here. You want to think through the deployment of agents from a human-centered standpoint, improving customer satisfaction and building trust along the way. This includes considerations around brand alignment — does the agent use the right tone, does it align to our value and principles, and is it an effective extension to create a personalized interaction.
To do this well, it requires extensive testing and feedback mechanisms that allow the agents and the digital labor it is performing to evolve.
It goes without saying that while most organizations are just entering the fray of deploying agents, they are struggling with the fact that most enterprise applications they have invested in have their own form of AI or agents. As such, they are struggling with questions around where I start, what do I build where, and how do I assess its success. These questions will likely become more apparent, but if we were to look slightly out to the near-term, the next big question could be “how I coordinate activities, processes, and workflows between agents (either within or between enterprise applications)”. This is one of those where the answer is not yet clear, but the manner and reason by which you build agents should factor in the reality of collaboration that will likely need to occur between agents. Can there be handoffs? Does the data set change? Is there a hybrid of assistive and anonymous?
I don’t venture to suggest the technology has even solved this yet, but again, the speed at which it is evolving is realistic to expect that the proliferation of agents will often require this coordination to increase the outputs.
The takeaway to the above points shouldn’t be “oh this is complex; we can’t do it”. As you look to deploy agents as digital labor, you set the appropriate expectations around skills, timelines, and inputs that are required to help drive a successful outcome. Like everything else, getting things done is not the same as getting things done right.
Finally, as referenced in this article, we should start getting on board with the concept that agents will likely be digital labor. As you should constantly reinvent your business model to remain relevant and align to the future of work, which requires constant upskilling of your labor, this same concept should be applied to digital labor; constantly helping them learn, enable, and upskill based on data, insights, and changes can be required. Organizations should capitalize on digital labor to lead, and if they wait too long, they may fall too far behind to catch-up.
Reach out to learn more about our AI Activation Path for Agentforce, where we’re guiding clients through their agentic journeys aligned to their current maturity level, data landscape, and industry with a high-impact agent use case deployed in five weeks.
PwC empowers organizations at every stage of their AI journey, delivering innovative customer experiences with AI-powered agents from Salesforce.
PwC can help you leverage the flexibility of Salesforce’s new consumption based pricing model while driving value with agentic AI.
Phygital service excellence provides a holistic approach to servicing a customer’s needs by delivering a consistent, data-driven, personalized experience.
What does it mean to supercharge your sales? PwC and Salesforce can help you reimagine your sales functions and fuel desired growth utilizing data and AI.