PwC’s strategic alliances
PwC's strategic alliances with a select number of companies allow us to better serve our clients and address the most critical business issues.
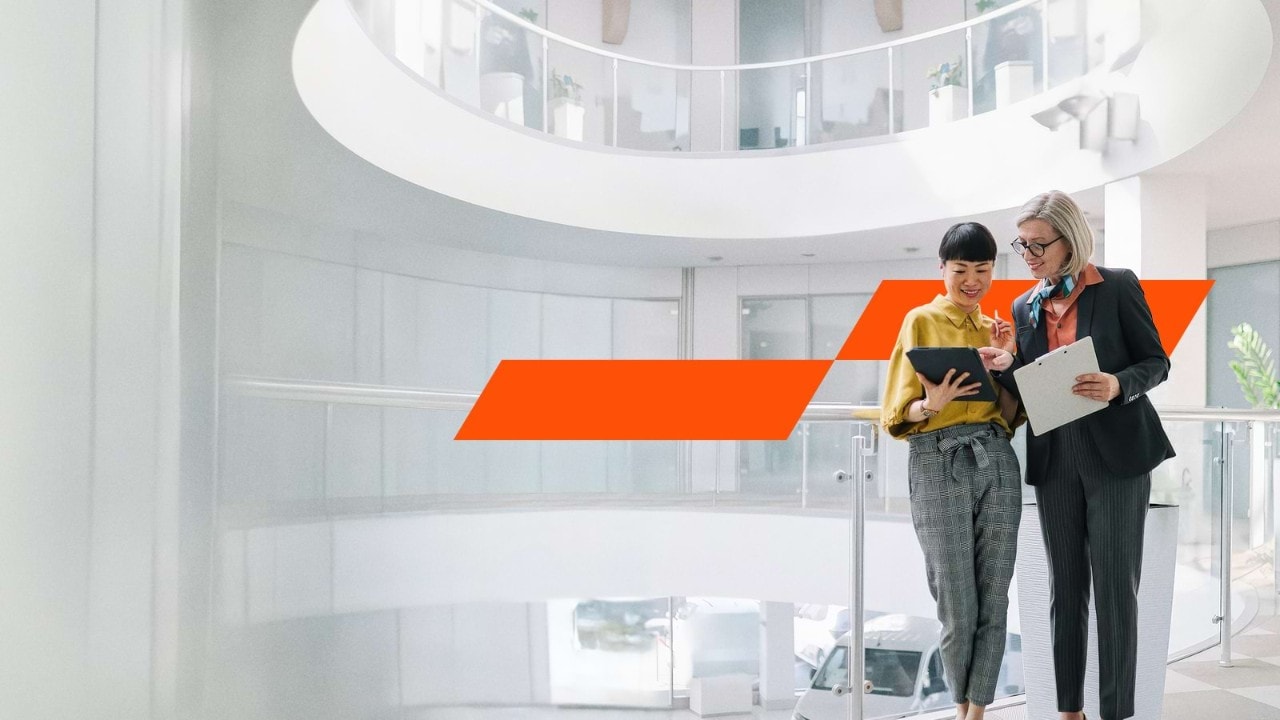
Make decisions with confidence and clarity to achieve your business objectives.
We help you cut through complexity — with strategy, speed and staying power.
When the pace of change won’t slow down, we help you stay ahead. Whether it’s transformation, technology, deals or risk, our consultants bring the insights and experience to help you move forward — so you can turn big goals into real-world results.
PwC's strategic alliances with a select number of companies allow us to better serve our clients and address the most critical business issues.
PwC's Analyst Relations Programme helps provide business, technology and industry analysts with insights into how we provide industry-focused services for private and public clients around the world.
How is next-generation cloud redefining outcomes and organizations? PwC’s 2024 Cloud and AI Survey reveals what it takes to compete in an AI economy.
AI is reshaping work—faster than ever. Discover how AI agents redefine workforce strategy, business models, and competitive advantage. Are you ready?
A practical guide for reimagining how your company creates, delivers, and captures value.
Explore PwC’s AI predictions with actionable strategies, industry insights, and trends shaping AI’s role in business transformation for 2025 and beyond.
PwC can help your company prepare for the SEC climate disclosure rules and other regulations that expand ESG reporting requirements.
Discover how your business can use AI to reshape business strategy, workforce and technology to drive success and gain a competitive edge.
Next Move discusses the latest regulatory and technology policy developments and how risk leaders can react. Read the latest issue on Responsible AI.
AI changes competitive advantage and revolutionizes business strategy. How to leverage data to transform the way you approach innovation and growth.
Tyson Cornell
PwC US Advisory Leader, PwC US
PwC US Chief Operating Officer of Advisory, PwC US