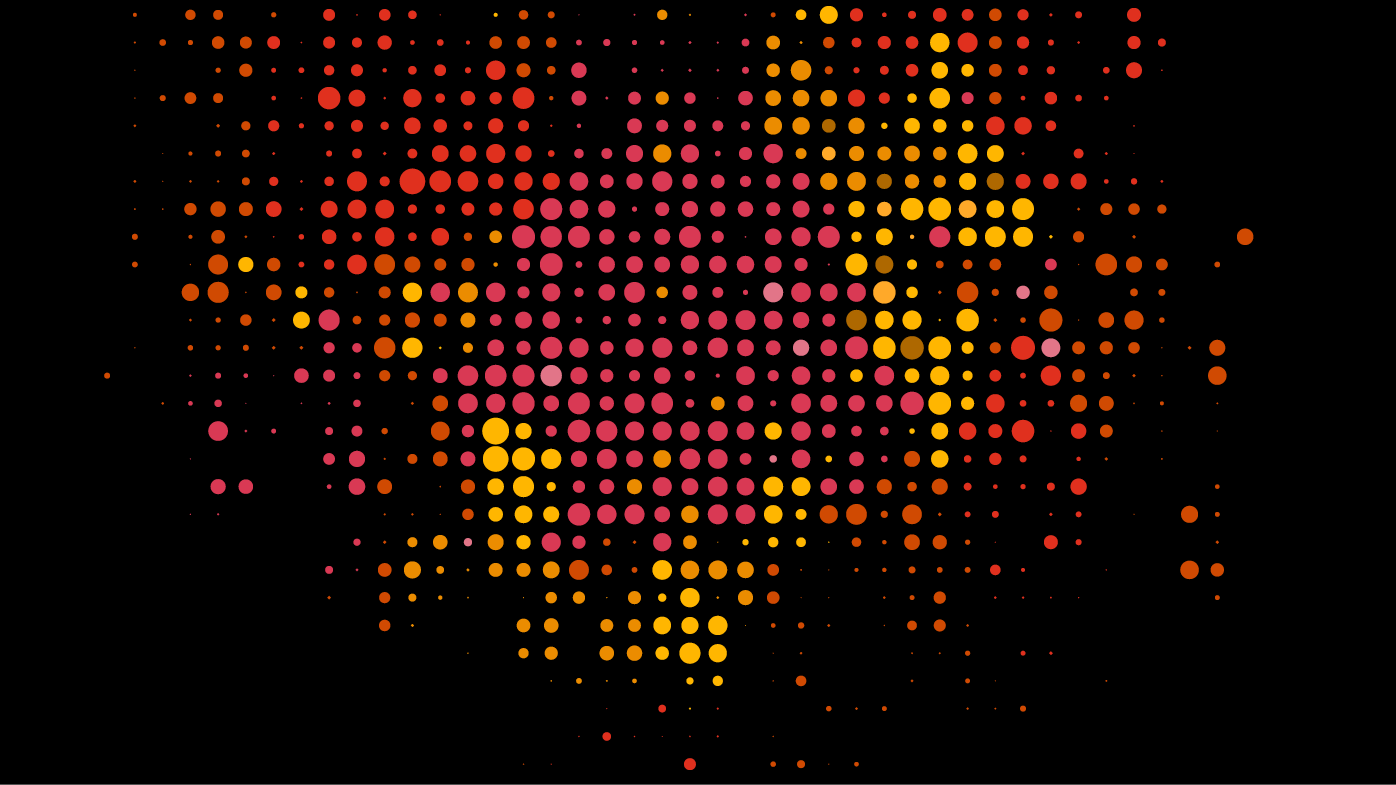
Generative AI is here
Explore how it can reinvent the way we work, accelerate innovation and drive trusted outcomes.
Learn moreIf you’re aiming to embed generative AI (GenAI) into operations, you may need to make changes to your AI playbook. Your current IT operating model is likely not set up to take advantage of GenAI’s potential for speed and scale. Instead, an “AI factory” approach can enable you to quickly start using a single GenAI model for multiple functions and tasks. The result can be both turbocharged productivity and efficiency right now — and new business models in the very near future.
At PwC, we know firsthand how to deploy generative AI at scale and manage the new risks it can bring. With the help of a three-year, $1 billion investment, we’re rolling out GenAI throughout our firm and helping our clients do the same. GenAI can have far-reaching benefits across your business, with high degrees of repeatability. Its implementation can be more effective not in discrete projects but as an enterprise capability. We bring this to life with a GenAI Factory. Here’s what to consider about setting up your own AI factory.
If you’re aiming to embed generative AI (GenAI) into operations, you may need to make changes to your AI playbook. Your current IT operating model is likely not set up to take advantage of GenAI’s potential for speed and scale. Instead, an “AI factory” approach can enable you to quickly start using a single GenAI model for multiple functions and tasks. The result can be both turbocharged productivity and efficiency right now — and new business models in the very near future.
At PwC, we know firsthand how to deploy generative AI at scale and manage the new risks it can bring. With the help of a three-year, $1 billion investment, we’re rolling out GenAI throughout our firm and helping our clients do the same. GenAI can have far-reaching benefits across your business, with high degrees of repeatability. Its implementation can be more effective not in discrete projects but as an enterprise capability. We bring this to life with a GenAI Factory. Here’s what to consider about setting up your own AI factory.
There’s a great way to achieve significant ROI quickly from generative AI. Take advantage of how a single, repeatable “pattern” of generative AI training and deployment can apply across your value chain. With conventional AI, you may have needed a different, custom-built AI model for every use case. With GenAI, a single pattern of deployment may be able to help produce or troubleshoot software code in many functions or lines of business.
Since generative AI models come “pre-trained,” requiring only adaptation and customization, you can often conduct a proof of concept quickly or even go straight to a pilot. Naturally, you won’t roll out GenAI everywhere all at once. You’ll start with a few use cases and learn from mistakes and successes. But with the right preparation, you can move far more quickly than with conventional AI. A 90-day “sprint” is often enough to get initial use cases up and running and ready to scale.
To create a roadmap for generative AI deployment, bring together technology and business leads. As you identify use cases, assess their value and look for patterns that can be replicated to deliver value elsewhere.
Often, a use case of moderate value that offers repeatability will deliver more ROI than a one-and-done high-value use case.
Identify data, technology and skills gaps and then determine the cost and timeframe for closing these gaps. Common gaps may include:
Your roadmap should also include trust-by-design — a plan to embed responsible AI from day one into the technology and processes needed to validate results, monitor ROI and manage and mitigate risks now and in the long term.
Several specialized companies, including the major cloud platform providers AWS, Google and Microsoft, as well as OpenAI, offer generative AI “foundation models” — deep learning algorithms pre-trained on massive amounts of public data. Once selected, you’ll then want to work with your cloud provider to establish a private version of the model within your firewall.
A private version of a foundation model can enable you to increase GenAI’s value by customizing it with your own data, context, intellectual property and expertise while maintaining proper security.
You can, for example, have your most experienced specialists embed your own data and IP to be used by a GenAI model. Then everyone in your organization will have access to their expertise — as if GenAI were providing them with personalized guidance from a mentor.
As you upgrade internal technology and cloud capabilities for GenAI, pay special attention to data. Generative AI can make sense of unstructured data, but you may still have to cleanse and organize at least some of it — mitigating, where appropriate, the risks of bias in data sets. You’ll likely also need to create data pipelines, complete with new APIs, to provide generative AI with continual access to the most up-to-date data available. To safeguard your data and intellectual property, you may also need to upgrade both data governance and cybersecurity for GenAI’s potential risks.
To make deployment and execution fast and repeatable, build an AI factory — an operating model built on pods to identify and assess use cases in a selected domain or line of business — and then adapt the foundation model to deliver value. Each pod contains six roles.
Staffing a GenAI factory will likely mean you may need to hire some specialists and upskill many more existing business and tech roles.
But GenAI’s remarkable scalability can help ease the demands on your workforce too. Technology specialists (such as model mechanics and data scientists) can usually work many pods at once. As we do at PwC, internally and with clients, you can also use pre-built toolkits with ready-to-use software code and prompts. These toolkits can help speed up the deployment and reduce the costs of GenAI for things like personalized customer experiences, content creation, research, agile software delivery, support services, report generation, deep retrieval, smart summaries and Q&A engines.
With your generative AI factory in production mode, your focus can now shift to trust and the continuous assessment of performance, risks and outputs. To address generative AI’s most important risks, PwC’s Responsible AI framework covers strategy, controls, responsible practices and core practices. It can be specially tailored to address GenAI’s greatest potential risks, including biased, offensive or misleading content; new challenges to interpretability; new cyber and privacy threats; and “hallucinations” that threaten performance.
Oversight is also about far more than risk management. It can also help you keep managing costs and growing value. A well-designed oversight framework — complete with comparisons with historical data sets and periodic audits by both domain experts and data scientists — can help you continually monitor effectiveness, costs, alignment with business objectives and overall ROI. You can then make changes as needed, backed up by verifiable data, to continually boost that ROI.
Generative AI is already transforming business. Contact us to learn more about this rapidly evolving technology — and how you can begin putting it to work in a responsible way.