The leader’s guide to value in motion
Get ready for AI, climate change and other megatrends to shift value pools, reconfigure industries and redefine the top management agenda.
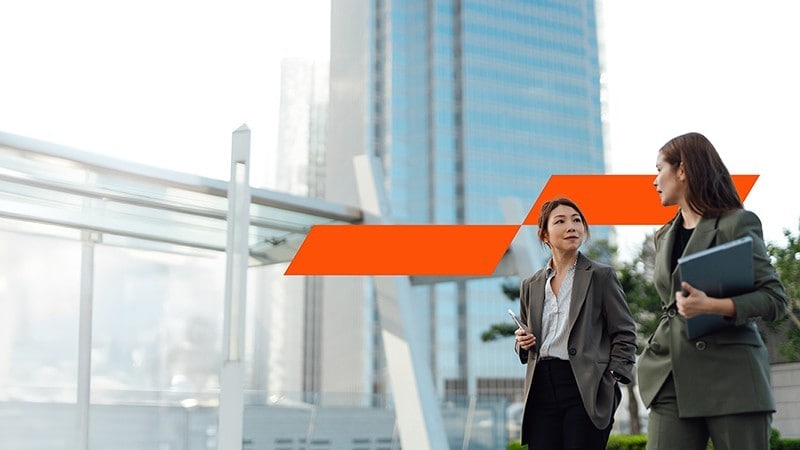
Powerful AI models have begun driving productivity gains and innovation at companies in every sector. At the same time, the boom in data centre construction has placed real strain on the energy system. By 2026, data centres worldwide, including those used to run AI, could use as much electricity as Japan, according to the IEAOpens in a new window. To meet this demand, tech giants, investors and energy companies have been teaming up on multibillion-dollar projects to add generation capacity—relying on fossil power in some cases, on nuclear in others. And some big tech firms with ambitious carbon-reduction targets have acknowledged that running AI models at scale has caused their emissions to spike.
It’s equally true that AI enables sustainability solutions. Companies can harness this game-changing technology to improve efficiency in a range of areas, especially energy use. Such applications, according to a report by PwC Germany, Microsoft and Oxford University include predicting when rooms will get hot and automatically pre-cooling them; optimising the routes of commercial aircraft and cargo ships; and refining the composition of cement and the processes used to make it.
Which raises a question: could AI compensate for its own heightened energy use and emissions footprint by finding and enabling efficiencies? PwC built a model to simulate AI’s effect on energy demand in two settings: data centres and the rest of the economy. And we found that if AI could improve energy efficiency across the economy at one-tenth of the rate of adoption, it would make up for the additional energy required by data centres—such that AI’s overall effect on energy use and emissions could be neutral.
Data centres have garnered a lot of attention in the discussion about AI and energy. These facilities are where most AI-related energy use takes place, because they house chips and hardware that perform the work of AI training and computations (along with other types of work, such as delivering cloud computing services). Tech companies have been busy setting up more and more data centres to meet demand for AI services; Microsoft has announced plans to invest some US$80 billion in data centres during fiscal 2025. And companies are expected to keep building them, as ever more people and organisations do more and more with AI.
Training and running AI models typically requires enormous resources—though recent reports about the model built by DeepSeek, a Chinese start-up, have focused attention on the possibility of engineering AI tools that can operate much more economically. Research has also shown promising routes for the development of more efficient chips and cooling methods for data centres. Even allowing for plausible improvements in the efficiency of AI software and hardware, the amount of energy used by data centres will likely increase. Our own modelling of three scenarios suggests that projected rises in AI use would cause data centres to consume 13–16% more energy in 2035 than in a baseline scenario where AI use is more consistent with current levels. Over the period 2024–35, total energy consumption in data centres would be 18–21% higher with extensive AI use than without it. (For more about our research approach, see the ‘About the analysis’ box at the end of this article.)
To model the impact of AI on the rest of the economy (that is, everywhere but data centres), we assumed that companies and organisations will use AI to improve energy efficiency, in the interest of saving money and reducing carbon emissions. Already, plenty of AI applications exist to help companies and organisations do just that. These can automatically adjust electric vehicle–charging patterns and fine-tune manufacturing processes, among other things. And there’s reason to think more applications will be developed: PwC finds that climate-tech start-ups working with AI raised US$6 billion of venture funding in the first nine months of 2024, which was US$1 billion more than they raised in all of 2023.
Assuming that each percentage-point increase in AI use leads to a 0.1 percentage point improvement in energy efficiency, directly or indirectly, projected increases in AI adoption would result in 0.3% to 1.3% less energy use outside data centres in 2035 than there would be with baseline levels of AI adoption. Over the period 2024–35, total energy savings outside data centres would be 0.1% to 1.0%.
Accounting for the rise of energy consumption in data centres and the potential AI-driven gains in efficiency, we find that AI adoption would reduce energy consumption across the economy to a modest degree. In the year 2035, the net reduction in energy used is 0.5% to 1.1%, depending on the scenario. And over the period 2024–35, AI adoption would result in cumulative changes in energy use that could range from a reduction of 0.9% to an increase of 0.1%. All told, the additional energy use in data centres would be roughly offset by the energy savings realised in the rest of the economy.
Finally, we estimated the difference in greenhouse gas (GHG) emissions, across data centres and elsewhere, associated with widespread AI adoption (and, in some scenarios, a more pronounced shift to renewable energy). These calculations show that widespread AI adoption could cut total GHG emissions for the period 2024–35 by 0.1% to 1.1%. And in 2035, emissions would be 0.3% to 1.9% lower with widespread AI adoption than without it.
Tech firms and data-centre operators aren’t the only businesses looking to take advantage of AI’s performance-boosting potential while managing its energy needs and climate impact. Any company that’s using AI applications—be it to enhance products or create new ones, provide better experiences to customers or streamline business processes—stands to benefit from optimising their energy demand. Experience suggests that four actions can help companies get the AI-energy equation right.
Use AI as a demand-side energy solution. Managing energy demand offers businesses a relatively straightforward way to trim costs and curb emissions. Research by the World Economic Forum in collaboration with PwC suggests that companies worldwide could save US$2 trillion per year by 2030 by making full use of currently available technologies. As the global energy system gets more digitised, companies will be able to supply AI models with more and better data that they can use to reap energy savings.
Track the emissions of your AI programme. At companies that rely on cloud-based services rather than on-site servers, AI usage generates Scope 3 emissions (those resulting from activities in an organisation’s value chain rather than its direct operations). The major cloud-AI providers now offer emissions trackers to their customers. Such trackers can help IT and sustainability teams evaluate the environmental performance of their AI initiatives and find ways of reducing associated costs to the business.
Right-size AI for business needs. Large language models (LLMs), for example, typically perform a given task at a higher cost and a greater energy expenditure than smaller models which are designed for that task. Choosing the right AI tool for each task, rather than a do-it-all model with too much capability, can help companies save money and prevent excess emissions—a bit like driving a small car instead of a heavy off-road truck to get around town.
Consider sustainability when choosing AI vendors. Companies that provide LLMs rarely publish details on their energy consumption and environmental performance, and metrics haven’t been standardised. Nevertheless, corporate AI and sustainability teams can work together to seek out AI vendors that disclose overall sustainability measures and demonstrate a commitment to environmental sustainability through the use of optimised algorithms and advanced hardware solutions, along with data centres that draw as much of their main power and backup power as possible from renewable energy.
The business landscape is changing rapidly. Imagining your company under three profoundly different scenarios will help you locate opportunity.
AI, climate change and geopolitical shifts are reconfiguring the global economy. We’ve mapped where value is moving over the next decade, so you can build a future-ready business to capture it.